A robust modeling approach for fatigue damage in composites based on bayesian model class selection
Abstract:
A Bayesian model selection approach is presented for selecting the most robust model class among a set of physics-based models for fatigue damage prognostics in composites. Three families of micro-damage mechanics models (shear-lag, variational and crack-opening displacement) are chosen to capture the relationship between stiffness reduction and matrix-cracks density in composite fiber-reinforced polymers (CFRP). First, the candidate models are parameterized by global sensitivity analysis, and then, they are ranked through probabilities that measure the extent of agreement of their pbkp_redictions with observed SHM data, while avoiding the extremes of over-fitting or under-fitting. These probabilities are computed based on the evidence provided by the data and the modeler's choice of prior probability for each model class. A case study is presented using multi-scale fatigue damage data from a cross-ply CFRP laminate. The result leads to the conclusion that, in this case, the most probable among competing models is found to be the model that has the lowest complexity. This principle, also known as Ockham's razor, seems to hold true for the composite materials investigated here because more complex models are adversely affected by statistical noise.
Año de publicación:
2014
Keywords:
Fuente:
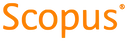
Tipo de documento:
Conference Object
Estado:
Acceso restringido
Áreas de conocimiento:
- Material compuesto
- Material compuesto
- Optimización matemática
Áreas temáticas de Dewey:
- Ingeniería y operaciones afines